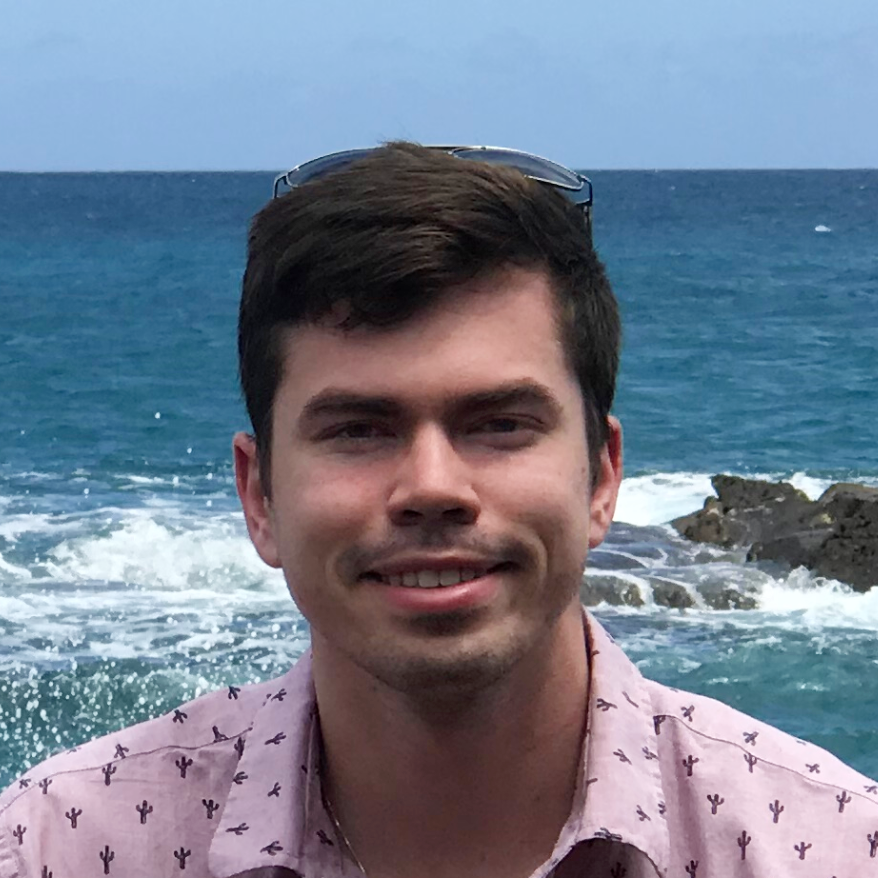
My Passion
driving innovative research at the intersection of physics and machine learning
My primary research interest is to integrate machine learning models with physics knowledge. More generally, I am interested in developing novel solutions to problems involving physical processes through the use of machine learning and probabilistic models.
I am a Ph.D. student at NYU researching experimental particle physics with a machine learning focus under the advising of Kyle Cranmer. In particular, I am working on realizing original applications for machine learning in particle physics analyses. Examples of such research by others include QCD-aware neural networks, using convolutional neural networks for jet reconstruction through jet images, and using generative adversarial networks for efficient simulations. As an undergraduate at Notre Dame, I double-majored in Physics and Honors Mathematics with a concentration in Advanced Physics and graduated with honors in each.
I want to know how God created this world. I'm not interested in this or that phenomenon, in the spectrum of this or that element. I want to know His thoughts; the rest are details.
- Albert Einstein